Noise-in, Bias-out: Balanced and Real-time MoCap Solving
Oct 3, 2023·
,,,·
1 min read
Georgios Albanis

Nikolaos Zioulis
Spyridon Thermos
Anargyros Chatzitofis
Kostas Kolomvatsos
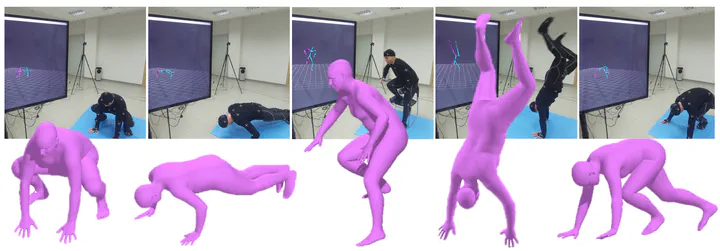
Abstract
Real-time optical Motion Capture (MoCap) systems have not benefited from the advances in modern data-driven modeling. In this work we apply machine learning to solve noisy unstructured marker estimates in real-time and deliver robust marker-based MoCap even when using sparse affordable sensors. To achieve this we focus on a number of challenges related to model training, namely the sourcing of training data and their long-tailed distribution. Leveraging representation learning we design a technique for imbalanced regression that requires no additional data or labels and improves the performance of our model in rare and challenging poses. By relying on a unified representation, we show that training such a model is not bound to high-end MoCap training data acquisition, and instead, can exploit the advances in marker-less MoCap to acquire the necessary data. Finally, we take a step towards richer and affordable MoCap by adapting a body model-based inverse kinematics solution to account for measurement and inference uncertainty, further improving performance and robustness.
Type
Publication
In 2023 IEEE/CVF International Conference on Computer Vision Workshops (ICCVW)
Click the Cite button above to copy/download publication metadata (*.bib).